Predictive Metabolomic Profiling of Microbial Communities Using Amplicon or Metagenomic Sequences
By Dr. Katie E Golden, MD
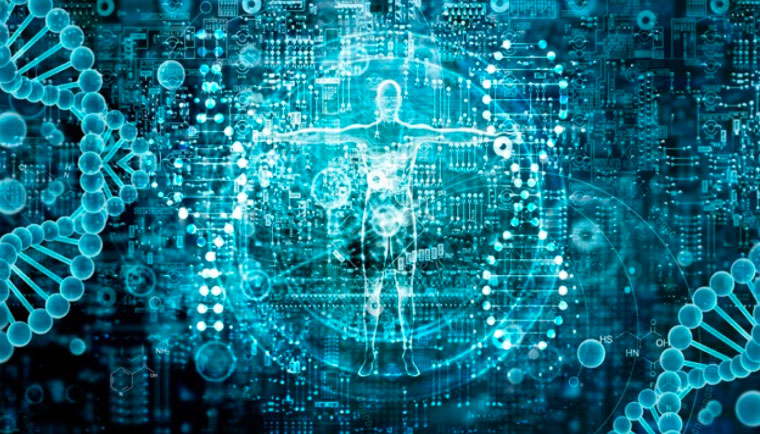
Microbiome researchers have become increasingly focused on how the small molecules produced by bacterial communities impact human metabolism. Metabolomics has taken us a step beyond simply defining the taxa that play a role in health and disease, and asks how the microbiome alters the biochemical pathways that drive homeostasis and dysbiosis. Large scale metabolomic analysis of intestinal bacteria, however, is both costly and logistically challenging. In a recent study, researchers have developed an innovative computer model to pair metabolomes with readily available metagenomic data to predict the metabolic activity of microbiome communities.1
In an article published in Nature Communications, Mallick et.al. unveil their computational framework MelonnPan (Model-based genomically Informed High-dimensional Predictor of Microbial Community Metabolic Profiles), which will allow future researchers to infer metabolic profiles of bacterial communities from metagenomic sequences. Metagenomic analysis of microbial communities is widely available, relatively cost-effective, and can be easily applied to a large scale of bacterial populations. The authors paired metagenomic and metabolomic datasets from Inflammatory Bowel Disease patients and healthy controls to train a computer model to identify the microbial features that were most predictive of metabolomic profiles. They then quantified the accuracy of the predicted metabolites. The result is a reproducible framework that can recover trends for more than 50% of community-associated metabolites.
While their novel approach does not replace metabolomic profiling, its time and cost efficient approach will be instrumental in guiding future investigations. To further enhance hypothesis generation and study design, the authors also created an accompanying confidence score that provides quantification of the models expected performance on a particular sample. MelonnPan users can use these values to infer how much additional data is needed to accurately characterize the metabolome of new bacterial communities. To test the utility of their new model across a variety of microbial communities, the authors applied their technology to paired metagenomic and metabolomic data sets from bacterial populations in three different environments: coral reefs, murine gut, and human vaginal microbiomes. They found that over 60% of the analysed metabolites were accurately predicted across all three data sets.
This novel model will help generate larger scale metabolomic data, which is a critical step in understanding the molecular pathways that define the host-microbe relationship. If we can uncover the bacterial-derived molecules that drive the pathogenesis of inflammatory conditions as well as the restoration of homeostasis, we can harness this knowledge to develop preventative and treatment strategies for microbiome-associated disease.
1. Mallick, H., Franzosa, E.A., Mclver, L.J. et al. Predictive metabolomic profiling of microbial communities using amplicon or metagenomic sequences. Nat Commun 10, 3136 (2019).